Generative AI and machine learning are engineering the future in these 9 disciplines
Generative AI vs Machine Learning
This is the concept we think of as “General AI” — fabulous machines that have all our senses (maybe even more), all our reason, and think just like we do. You’ve seen these machines endlessly in movies as friend — C-3PO — and foe — The Terminator. General AI machines have remained in the movies and science fiction novels for good reason; we can’t pull it off, at least not yet.
We’re interested in both and the future will be a hybrid of both,” Altman said when answering a question about small, specialized models versus broad models during a Q&A session at Station F earlier this year. “ZenML is sort of the thing that brings everything together into one single unified experience — it’s multi-vendor, multi-cloud,” ZenML CTO Hamza Tahir said. The reason ZenML is interesting is that it empowers companies so they can build their own private models.
The Pros and Cons of Machine Learning
We might be able to write enough rules that our app could successfully identify whether or not something was a dog most of the time—but there would always be something we forgot. Mechanical engineering shapes everything from the vehicles we drive to the appliances we use at home. It encompasses designing, analyzing, and manufacturing various mechanical systems, from simple mechanisms, such as levers and pulleys, to complex machinery like aircraft engines and robotic arms. Although these are two related technologies and sometimes people use them as a synonym for each other, but still both are the two different terms in various cases. Let’s understand Machine Learning more clearly through real-life examples.
Some technology that was once revolutionary AI is now considered basic computer functions, and that trend of technology growth is likely to continue. Data managers or data scientists help utilize AI and develop ways to keep the data secure and available for us to use. AI research involves helping data-driven machines learn how to take new data as part of their learning problem and solution process. Artificial intelligence, the broadest term of the three, is used to classify machines that mimic human intelligence and human cognitive functions like problem-solving and learning.
What are the advantages and disadvantages of machine learning?
Even this example is getting ahead of itself, because until recently neural networks were all but shunned by the AI research community. They had been around since the earliest days of AI, and had produced very little in the way of “intelligence.” The problem was even the most basic neural networks were very computationally intensive, it just wasn’t a practical approach. Machine learning is a relatively old field and incorporates methods and algorithms that have been around for dozens of years, some of them since the 1960s. These classic algorithms include the Naïve Bayes Classifier and the Support Vector Machines, both of which are often used in data classification.
In that product, you now have pre-written answers for customer support interactions. It can compose business letters, provide rough drafts of articles and compose annual reports. It can also compose novels – although the results may not be entirely satisfactory. Generative AI is used to augment but not replace the work of writers, graphic designers, artists and musicians by producing fresh material.
Finally, ML models tend to require less computing power than AI algorithms do. This makes ML models more suitable for applications where power consumption is important, such as in mobile devices or IoT devices. The examples of both AI and machine learning are quite similar and confusing. They both look similar at the first glance, but in reality, they are different. In turn, a favorable shift has occurred in AI and machine learning employment trends. The U.S. Bureau of Labor Statistics projects that the employment of data scientists will grow 36% from 2021 to 2031.
Put simply, AI is any process that allows computers to simulate human intelligence. Famously, British computer pioneer Alan Turing’s imitation game (now called the Turing Test) judged whether a machine could demonstrate learned behavior through
natural language responses to human-generated questions. Artificial intelligence could potentially be achieved through a variety of techniques like formal logic and decision trees.
Time Series Forecasting
Read more about https://www.metadialog.com/ here.
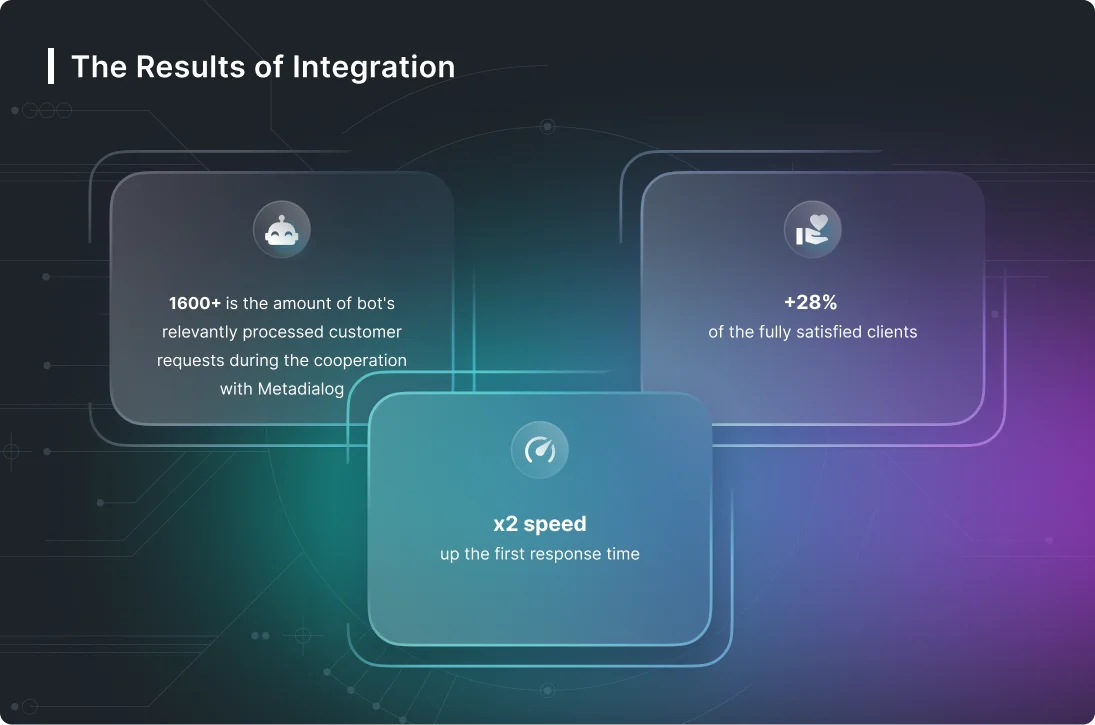